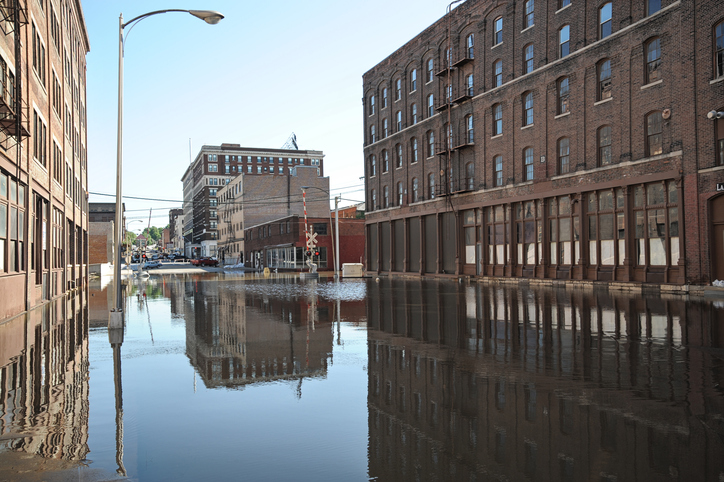
A New Approach to Anticipating Flood Damage
Max Dorfman, Research Writer, Triple-I (03/17/2022)
A recent research paper presents a “proof of concept” for predicting flood damage probabilities – using only open-source data and machine learning methods – that the authors say can “fill in gaps of unreported or unaccounted flood damage, identify unexpected damage, and rapidly update estimates [of flood damage probabilities] as new information becomes available.”
“This is the first spatially complete map of flood damage probability for the United States” said Ross Meentemeyer, professor of geospatial analytics at North Carolina State University Center and an author of the paper, adding that the information it provides can be used to learn more about flood risk in vulnerable, underrepresented communities.
Spearheaded by Elyssa Collins, a doctoral candidate in the North Carolina State University Center for Geospatial Analytics, the research used machine learning – a type of artificial intelligence that employs algorithms to automatically update data, in effect training the program. Inputs included flood severity, climate, and socio-economic exposure, among others.
Better data key to resilience
About 90 percent of all U.S. natural disasters involve flooding. Improved decision-making around mitigation and resilience requires improved data.
“Whether it’s building codes or pre-emptive risk mitigation, it costs money,” said Dr. Michel Léonard, Triple-I’s chief economist and data scientist. “You have to have people ultimately say, ‘It’s worth the money.’ The better the data at your disposal, the more accurately you can justify the expense.”
The study uncovered areas where the flood damage probability was greatest, exposing the potential for floods common in both inland and coastal regions. Flood damage probabilities trended in low elevation areas, close to streams with extreme precipitation, and was also greatly affected by high urban road density. Researchers found a high likelihood of flood damage with possible monetary damage, human injury and loss of life for over a million square miles across the country over the period studied.
In contrast to Federal Emergency Management Agency (FEMA) maps, 84.5 percent of the damage reports analyzed fell outside FEMA’s high-risk flood areas. Of this, 68.3 percent were found to be outside the high-risk floodplain, with another 16.2 percent uncharted by FEMA.
“We’re seeing that there’s a lot of flood damage being reported outside of the 100-year floodplain,” Collins said.
More to accomplish
According to a 2020 report from the Association of State Floodplain Managers, FEMA has spent up to $11.8 billion to create national Flood Insurance Rate Maps, which show whether an area has at least 1 percent risk of flooding in a year. Machine learning could approximate flood maps more expediently as circumstances change or as more data is mined.
“There is still work to be done to make this model more dynamic,” Collins added. “But it’s part of a shift in thinking about how we approach these problems in a more cost-effective and computationally efficient manner.”